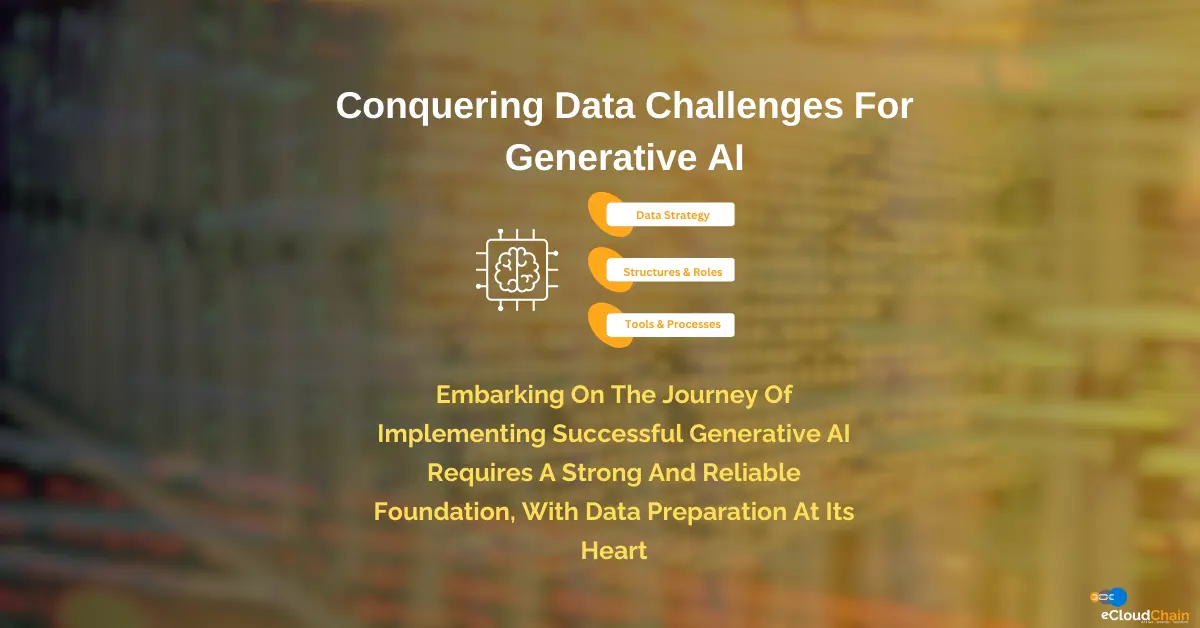
Conquering Data Challenges For Your Generative AI Success
Embarking on the journey of implementing successful Generative AI requires a strong and reliable foundation, with data preparation at its heart.
One of our enterprise customers encountered significant challenges with poor query performance, high maintenance costs, and a lack of centralized data governance. We addressed these issues by building a comprehensive data lake using AWS services such as AWS Lake Formation, DMS, S3, Apache Iceberg, AWS Athena, and AWS Glue. This solution significantly improved query performance, reduced maintenance costs, and established centralized data governance. This success story demonstrates how our data integration with AWS services can transform enterprise data management.
Customer faced significant challenges with data and infrastructure management on their on-premises setup. They struggled with scalability, high costs, limited skilled resources, and a lack of visibility on future strategies. These issues resulted in lost business to competitors.
They urgently needed to address these critical problems, starting with consolidating their data and improving data management. In summary, their data management issues included:
The challenges faced by the customer resulted in high costs due to ineffective data management. Data silos led to inefficiencies as stored data lacked integration, causing redundant storage costs without yielding meaningful insights. The organization lacked visibility into its data landscape, hindering decision-making and strategic planning.
These issues highlighted the need for a centralized data governance approach to optimize storage, integrate diverse data types, and unlock actionable insights. By addressing these challenges, the customer aimed to enhance operational efficiency and leverage data effectively for informed decision-making and future growth initiatives.
These initiatives aimed to prepare the organization for future AI/ML capabilities, leveraging the centralized data lake infrastructure for various advanced analytics and machine learning use cases.
Our team chose Amazon S3 for storing data in a structured Apache Iceberg table format to ensure efficient management and scalability. To address the security challenges, we used AWS Lake Formation to provide secure access controls and manage data security across the platform. AWS Glue was used to handle the Extract, Transform, Load (ETL) process, seamlessly preparing data for analysis and storage in the data lake. Athena was implemented as the query engine, allowing SQL-based queries on the latest inventory data stored in Iceberg tables.
This comprehensive setup ensures robust data governance, scalability, and agility in data processing and analytics with following workflow:
Embarking on the journey of implementing successful Generative AI requires a strong and reliable foundation, with data preparation at its heart.
No AI strategy can thrive or endure without high-quality data because data is the lifeblood that fuels generative AI…
Amazon Athena lets you query data where it lives without moving, loading, or migrating it. You can query the data from relational, non-relational…
Amazon Redshift is a cloud-based next-generation data warehouse solution that enables real-time analytics for operational databases, data lakes….