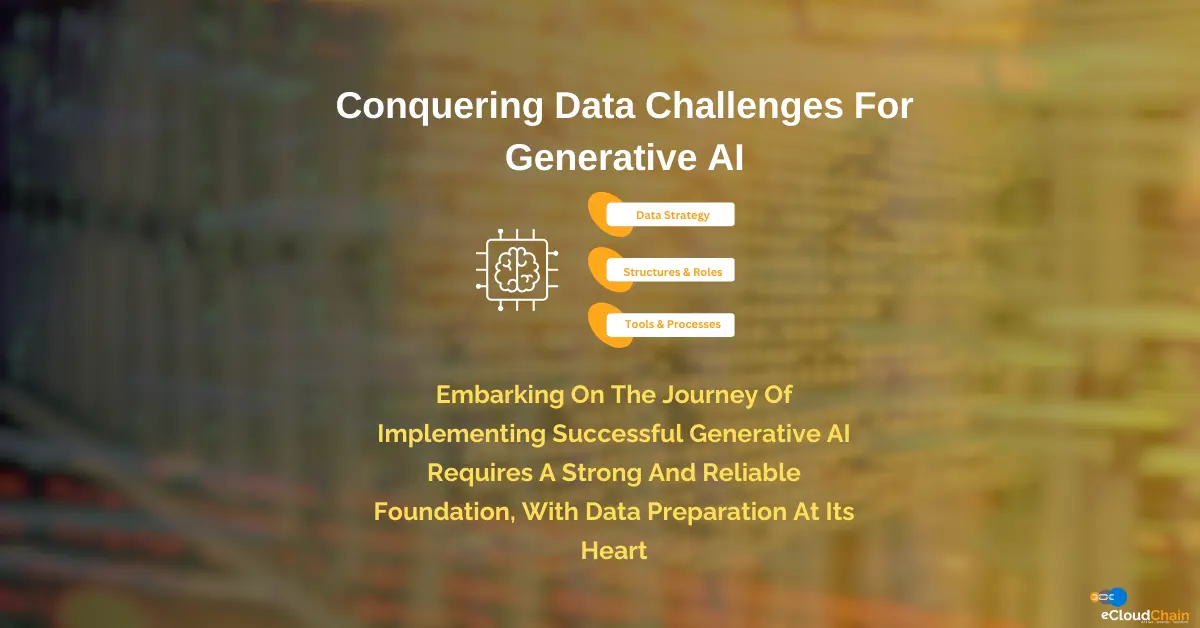
Conquering Data Challenges For Your Generative AI Success
Embarking on the journey of implementing successful Generative AI requires a strong and reliable foundation, with data preparation at its heart.
Data Preparation and Pipelines: Enterprises struggle with preparing data and building efficient data pipelines on-premises due to the complexity and cost of infrastructure setup and maintenance.
Limited Skilled Resources: There is a shortage of skilled professionals to build and maintain cloud infrastructure, and to continually cleanse and manage diverse data sources and pipelines.
Data Silos and Integration: Data sources are scattered across silos, making it challenging to streamline and integrate them into a cohesive data warehouse, hindering the ability to derive meaningful insights.
A leading B2B manufacturing customer approached eCloudChain to overcome challenges related to cost, skillset, and know-how in managing their data. Our team, with their agile approach, collaborated closely with the customer to address these issues. By building a centralized data lake, we provided the customer with a unified repository, enabling better access to data and the ability to process meaningful insights efficiently. This centralized solution not only reduced costs but also empowered the customer’s team with the necessary skills and expertise to leverage their data effectively.
Cost Efficiency: The customer aimed to reduce the high costs associated with managing and maintaining their data infrastructure in-house, seeking a more economical solution through subscription-based analytics services.
Skillset Enhancement: The customer needed access to advanced data analytics skills, which were lacking in their existing team, to effectively manage and analyze their diverse data sources.
Knowledge Improvement: The customer wanted to leverage expert know-how to streamline their data preparation and analysis processes, ensuring accurate and actionable insights without the steep learning curve.
Our data expert team collaborated closely with the customer to collect data from various sources, subsequently preparing and ingesting it into our AWS account using a managed model.
The team developed a robust and automated data pipeline to efficiently collect, clean, and prepare the data. Leveraging Amazon Redshift, S3, QuickSight, AWS Lambda, and other cloud and infrastructure services, we built multi-tenant applications ensuring data isolation, integrity, and confidentiality. This solution includes advanced features such as row-level security (RLS), column-level security (CLS) for fine-grained access control, role-based access control (RBAC), and permission assignment at the database and schema levels.
Additionally, our team pulled data from the customer’s operational databases, files, and APIs, ingesting it into the Redshift data warehouse. Data processing jobs were then utilized to enrich the data within Amazon Redshift. For comprehensive data processing and transformation, we employed Amazon Elastic MapReduce and AWS Glue to run Spark applications, ensuring efficient and scalable data management.
Embarking on the journey of implementing successful Generative AI requires a strong and reliable foundation, with data preparation at its heart.
No AI strategy can thrive or endure without high-quality data because data is the lifeblood that fuels generative AI…
Amazon Athena lets you query data where it lives without moving, loading, or migrating it. You can query the data from relational, non-relational…
Amazon Redshift is a cloud-based next-generation data warehouse solution that enables real-time analytics for operational databases, data lakes….